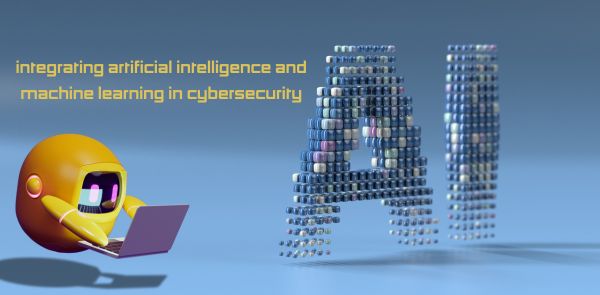
The age-old cat-and-mouse game between defenders and attackers has reached a new pinnacle with the integration of Artificial Intelligence (AI) and Machine Learning (ML). These technologies are no longer just buzzwords; they are reshaping how organizations approach cybersecurity, making defenses more proactive, adaptable, and intelligent. For cybersecurity experts, understanding and implementing AI and ML strategies can mean the difference between a resilient infrastructure and one prone to breaches.
The Current Cybersecurity Landscape
The increasing sophistication of cyberattacks—ranging from advanced phishing schemes to complex ransomware—is outpacing traditional security measures. Manual processes, reactive monitoring, and signature-based detection systems struggle to keep up with the pace of modern cyber threats. Enter AI and ML: technologies designed to process vast amounts of data, recognize patterns, and identify anomalies, providing a robust, adaptive, and scalable approach to cybersecurity.
How AI and ML Transform Cybersecurity
- Threat Detection and Response
Enhanced Threat Detection: AI-powered security solutions can sift through millions of data points in real-time, recognizing patterns indicative of potential cyber threats. ML algorithms can learn from historical data to identify anomalies that traditional systems might overlook.
– Predictive Analysis: ML models can predict potential vulnerabilities by analyzing historical data on past attacks. This predictive approach allows cybersecurity teams to address issues before they escalate into full-blown incidents.
- Incident Response Automation
– Efficient Response Mechanisms: AI can be integrated into Security Orchestration, Automation, and Response (SOAR) platforms to automate low-level incident response tasks. This automation helps security teams focus on complex issues that require human intervention, optimizing resource allocation and reducing response times.
– Intelligent Threat Mitigation: AI-driven systems can autonomously initiate containment measures such as isolating affected systems, blocking malicious IPs, or disabling compromised user accounts based on predefined rules.
Strategies for Implementing AI and ML in Cybersecurity
- Building a Data-Driven Security Framework
– Data Collection and Management: For AI and ML to be effective, organizations must prioritize collecting clean, high-quality data. This includes data from network traffic, user behavior, application logs, and more. Investing in data management practices ensures that the models have a solid foundation to learn from.
– Continuous Model Training: ML models should be trained and updated frequently with new data sets to keep up with the rapidly changing threat landscape. Regular updates enhance the accuracy of detection and reduce false positives and negatives.
- Adopting Hybrid Solutions
– Combining AI with Human Expertise: While AI can process data faster than any human, it is not infallible. A hybrid approach that pairs automated threat detection with human oversight helps balance speed with critical thinking. Security analysts can validate and act on AI-generated insights to ensure comprehensive threat mitigation.
– Human Feedback Loops: Incorporating expert feedback into the ML training process can improve the accuracy of predictions and enhance the system’s adaptability to new threats.
- Leveraging AI for Threat Hunting
– Proactive Threat Hunting: With ML models continuously analyzing network activity, organizations can identify potential threats before they escalate. Automated threat-hunting tools can spot suspicious activities that human analysts may miss, such as lateral movement or unauthorized privilege escalations.
– Behavioral Analytics: AI-powered solutions analyze user behavior over time to detect anomalies. For instance, if a user’s access pattern changes drastically, it may trigger an alert for further investigation. This behavioral approach is crucial for identifying insider threats.
Overcoming Challenges in AI-Powered Cybersecurity
- Balancing Automation and Oversight
– Avoiding Over-Reliance: While automation can significantly reduce the workload for cybersecurity teams, over-relying on AI may lead to complacency. Regular audits and manual reviews ensure that automated decisions align with organizational policies and ethical standards.
– Combating AI-Driven Attacks: Just as defenders use AI, attackers are also leveraging it to develop more sophisticated attacks. Training security teams to anticipate AI-driven threats and adapt defenses accordingly is essential for staying ahead.
- Addressing Bias and Model Limitations
– Diverse Training Data: Ensuring that training data is representative of diverse attack types and environments minimizes biases in the model’s decision-making process. A biased model may overlook certain threats or produce skewed results.
– Transparency and Explain ability: Implementing AI solutions that offer explain ability helps security teams understand the logic behind decisions. This transparency is crucial for trust and for refining algorithms over time.
Best Practices for Cybersecurity Experts
Continuous Learning and Upskilling: AI and ML are ever-evolving fields. To stay updated with the latest tools and methodologies, cybersecurity professionals should pursue training and certifications in AI-focused cybersecurity courses.
– Collaborative Approach: Working closely with data scientists and AI engineers can help security experts build tailored solutions that address specific threats unique to their industry or organization.
– Investing in Comprehensive Tools: Implementing solutions that integrate seamlessly with existing security infrastructures, such as SIEM (Security Information and Event Management) and endpoint detection and response (EDR) platforms, enhances overall cybersecurity posture.
The Road Ahead: AI as a Cybersecurity Essential
AI and ML are revolutionizing how organizations think about and implement cybersecurity. They offer a way to shift from reactive to proactive defense strategies, improving response times, enhancing threat detection accuracy, and automating routine tasks. However, for cybersecurity experts, understanding the limitations, ensuring transparency, and maintaining a balance between human oversight and automated processes are essential.
Organizations that integrate AI and ML thoughtfully will find themselves better prepared to navigate the complexities of the modern threat landscape. By adopting these technologies strategically, cybersecurity professionals can secure not just their networks, but their place at the forefront of innovation in the industry.
The integration of AI and ML in cybersecurity is not just an advancement; it is a necessity. With cyber threats evolving at an unprecedented pace, leveraging the capabilities of AI and ML can provide organizations with the edge they need to stay protected. Embracing these technologies, while remaining vigilant to their challenges, ensures a resilient, forward-thinking security posture ready to meet the demands of today and tomorrow.